ExTech Landscape: The State of Conversational AI for the Enterprise
Report Authors: Charles Araujo and Dr. Frank Granito
Published in Partnership with The Institute for Digital Transformation
The State of Conversational AI for the Enterprise
Artificial intelligence (AI) has captured the imagination of the world. And if you listen to all the hype, it now appears to power nearly every facet of our existence.
Of course, this is far from the truth. Still, it’s unquestionable that various forms of AI are now seeping into vast swaths of the enterprise technology landscape with the promise of improved efficiency, faster throughput, and improved experiences.
However, AI isn’t a single technology.
Rather, it is a collection of related technologies that all hope to leverage machine intelligence in some way or another. Among this collection, one of the most impactful — and visible — forms of AI, in both a consumer and enterprise context, is so-called conversational AI.
This particular form of AI attempts to mimic the conversational abilities of humans to enable a user to interact with a computer in much the same way they might interact with a human. This technology, therefore, is particularly useful in use cases such as support, processing requests, and performing routine transactions (e.g., banking transfers, vacation requests, etc.).
Because of its high degree of utility in both improving workforce efficiency and in potentially improving the customer and employee experience, along with its high level of visibility as a primary engagement touchpoint, conversational AI has seen dramatic levels of investment and focus.
For these reasons, enterprise organizations are eager to leverage this emerging technology.
Conversational AI and The Digital Experience Value Engine
Despite its growing power, conversational AI is still not an end unto itself. Like all technologies, its power derives from an organization’s ability to leverage it to meet its strategic goals and objectives.
Therefore, to understand the value that conversational AI may offer the enterprise, it is necessary to assess it through a prism of defined business value. We believe the best way of doing so is to use what we call The Digital Experience Value Engine.
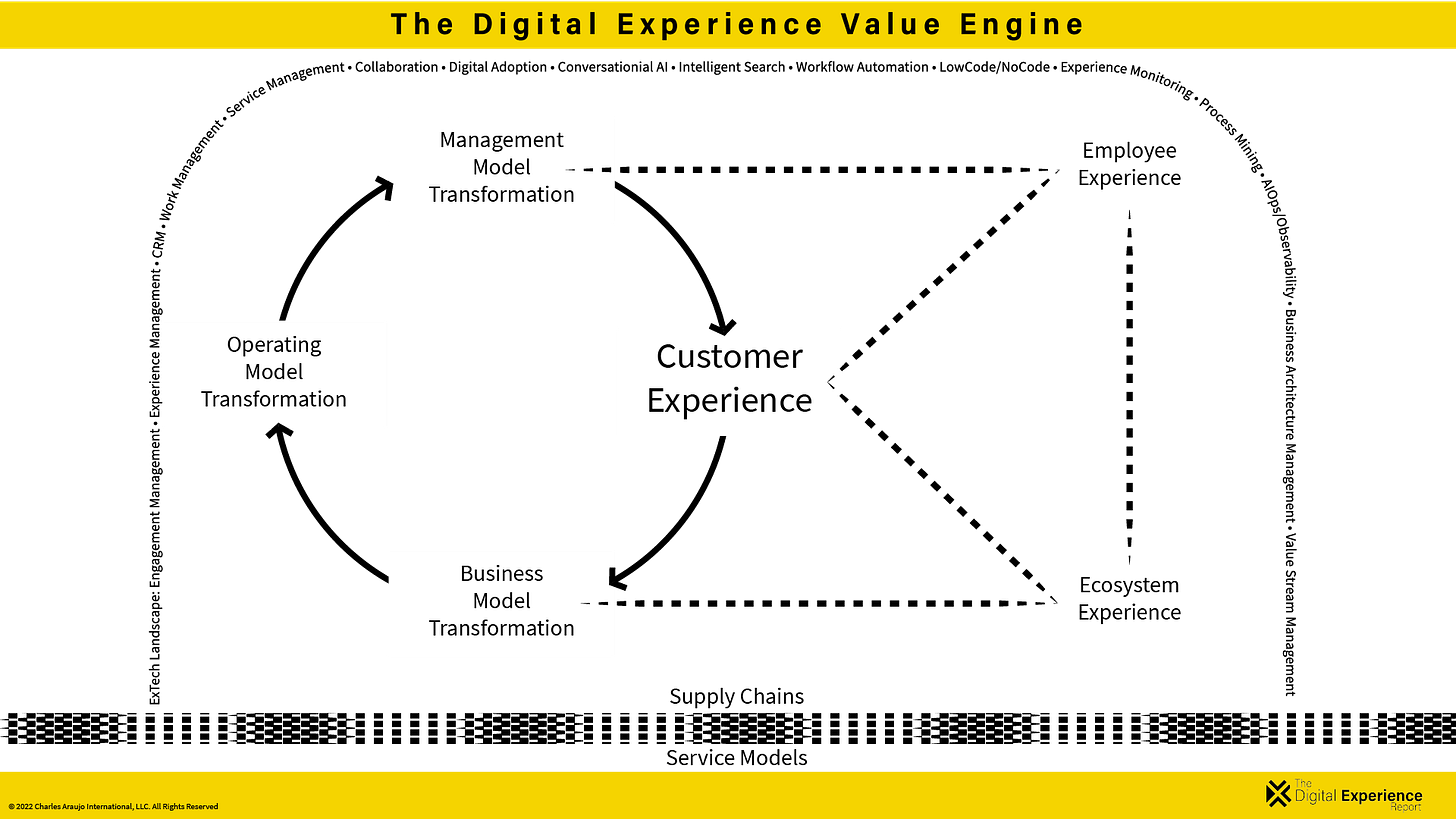
As defined in our previous research, Digital Experience Supply Chain: Reimagining Value Creation for the Digital Era, the nature of value creation in the enterprise has shifted away from optimization and efficiency as the primary drivers of value and to the creation and curation of a differentiated customer experience.
As such, organizations have been in a mad rush to reimagine the experience they deliver for their customers (and employees) and must now evaluate every technology investment they make through this prism.
Seen from this perspective, conversational AI provides significant potential value to the enterprise. If providers of this technology can deliver on its promise, it has the potential to help enterprises create highly personalized, contextual, and efficient experiences for their customers and teams, while simultaneously improving operational efficiency and throughput.
Moreover, because of its highly visible nature, conversational AI technologies also have the potential to be a catalyst, opening the door to even more profound business, operating, and management model transformations that enterprises will require to fully reap the benefits of adopting the Digital Experience Value Engine.
The purpose of this report is to help enterprise leaders understand the state of this pivotal technology sector, its various components, and how to best assess investments in these technologies.
The State of the Conversational AI Market
Part of what makes conversational AI so challenging for enterprise leaders is that it is not a one-dimensional sector. Technology providers can apply the underlying technology in a number of ways and to meet the needs of several use cases.
Therefore, we expect that most enterprises will deploy multiple forms of conversational AI against various use cases — a trend we observed taking shape during this research.
Likewise, the history of this class of technology has fed further confusion into the space. To therefore understand the state of the conversational AI market, we must examine its history and deconstruct it into its various market components.
The History of Conversational AI (and the Chatbot vs. Conversational AI Question)
The idea of conversational AI dates to at least the 1950’s with the advent of the so-called Turing Test.
In his seminal paper, Computing Machinery and Intelligence, Alan Turing posed the question of whether a machine could think and created the imitation game as a way to test his hypothesis. In it, he basically proposed what we would now consider to be a conversational AI agent to play the game in an attempt to convince a human interrogator that it was a human player.

Alan Turing. Unknown author. "Public Domain" according to Wikimedia Commons
In the over seventy years hence, computer scientists have been developing and testing various forms of technologies that could mimic a human conversation. Most of these early efforts created what we refer to as chatbots.
While there is little definitive clarity on the difference between chatbots and what we see as conversational AI, we do not believe they represent the same class of technology (or capabilities) and that the terms should not be used interchangeably (although several of the technology providers covered in this research do so).
We view chatbots as more simplistic, primarily relying on rules-based approaches and decision trees to govern their interactions. This approach makes them inherently less capable, less adaptable, and ultimately more likely to reduce efficiency and degrade the customer/employee experience rather than enhance either of them.
Conversational AI systems, on the other hand, leverage and combine multiple underlying AI technologies, such as machine learning (ML), natural language processing (NLP), and deep learning (among others) to create more natural, dynamic, and resilient interactions with their users.
Put simply, most chatbots will be unable to realize efficiency gains except in very narrow use cases and will almost always result in a negative user experience, effectively becoming the modern version of interactive voice response (IVR) systems that have been the bane of callers for years.
On the other hand, Conversational AI systems have the potential to serve a broader range of use cases and improve the customer and employee experience.
That said, the success of conversational AI platforms in delivering on this promise hinges on their ability to combine the underlying technologies in a way that sufficiently reduces training overhead and user friction. The proper application of a given technology against the targeted use cases and deployment channels will dictate much of this success or failure.
Classes of Conversational AI Technologies
One of the first things that an enterprise buyer needs to understand when evaluating conversational AI systems is that there are (at least) three primary classes of the technology. These classes are not good or bad, but do serve different purposes. The three primary classes of conversational AI technologies are:
Conversational AI Development Platforms
Consider these the building block players. They include platforms such as Microsoft’s LUIS, Google’s DialogFlow, and Amazon’s Lex (among others). These platforms are best thought of as developer tools and are used by both enterprises and other tech companies to develop conversational AI engines, but they do not offer an out-of-the-box experience. As foundational platforms they have expansive uses that extend far beyond enterprise use cases. Therefore, we did not include them in this analysis.
Conversational AI Wrappers
This class of technology essentially takes various forms of available conversational AI platforms and wraps them in an easy-to-use layer (sometimes adding proprietary technology in this layer) to meet the needs of specific use cases or industries. In most cases, the underlying component AI technologies are not proprietary to the technology provider.
Proprietary Enterprise Conversational AI Platforms
These platforms have been built using all (or mostly) proprietary AI technologies. Importantly, these platforms are typically built for one or more enterprise-specific use cases, which often drove the development or refinement of the underlying AI technologies.
Again, these classes do not represent good or bad, but it is important to understand the implications of each approach to your intended use case. Development platforms, for instance, offer you the greatest flexibility in creating AI-based solutions that may create a defensible competitive advantage, but will also require significant investments and resources to build and sustain.
Likewise, proprietary enterprise-focused technologies may offer greater efficacy for a specific use case, but is also dependent on the ability of a tech provider to keep up with AI advancements at pace with the tech giants.
We expect that most enterprise leaders will end up utilizing multiple classes of conversational AI technologies to solve for specific use case needs.
Deployment Channels
In addition to the technology classes, conversational AI platforms use various deployment channels. That said, two deployment channels are most widely used: text and voice.
It is clear that some users prefer to interact via text and others prefer to interact via speech. Nevertheless, the question of whether users really perform better while interacting with their preferred modality is an open question and one that is more probably concerned with preference or learning style. Moreso than with the classes of conversational AI, the deployment channels are not mutually exclusive, with many providers supporting both of them.
Still, it is important to understand your needs and your users’ preferred modality as these may impact your choices. These two deployment channels are characterized by:
Text centric channel
This is where all interactions and “conversation” is conducted through text. This channel includes such technologies as website-based chatbots, conventional text (e.g. SMS), or delivery through social channels such as WhatsApp or Slack.
Voice centric channel
This is where the interaction is conducted through text-to-speech and vice versa. This channel takes the form of digital assistants (e.g. Alexa, Siri, etc.), call center virtual agents, and also avatars which use voice in combination with a virtual humanoid figure.
We should also note that several technology providers drew a distinction between web-based text interactions and interactions via SMS or social channels. While we found that these distinctions had merit, the fundamental nature of text-based interactions are consistent across the various sub-channels. Furthermore, if a provider delivered effective interactions in one text-based medium, it was likely to do so in all others.
Still, if your primary use case is to interact with a specific constituency via a specific sub-channel (such as Facebook Messenger or WhatsApp), the effectiveness of a provider’s capabilities for that specific sub-channel would be germane, particularly in its ability to adapt to the specific constraints of that channel. For most use cases, however, these distinctions are nominal and a point of general parity between most providers.
Broad-based vs. Targeted Use Cases
It’s also important to note the distinction in use case applicability. Some providers of conversational AI have elected to deliver a broad-based solution that organizations can apply across a wide spectrum of use cases. Others have elected to focus on narrower sets of use cases or constituencies.
Those that have elected to focus on specific user constituencies or use cases claim that by doing so they are able to improve the quality of the conversational agents for those use cases or users. That seems logical, but the degree of improvement or its applicability must be evaluated based on your specific needs.
On the other hand, those providers that deliver a broad-based technology claim that it provides distinct advantages. For instance, some feel that the need to have a unified customer and employee experience dictates that you should have a single conversational AI platform to enable it. Others simply believe that the underlying technology is, by its nature, homogeneous and that there is little reason that it cannot be applied across a wide range of use cases.
Our analysis showed that there is no definitive answer to this question. Those vendors who specialize in targeted use cases or domains did demonstrate how that focus could decrease learning time and improve intent recognition. Still, those demonstrations seemed relatively nuanced and many of the broad-based approaches demonstrated compelling abilities to serve multiple use cases. Ultimately, enterprise leaders must assess the question of broad or narrow use based on their specific needs.
The Sophistication Conundrum
There was an additional finding that we found somewhat perplexing and have dubbed it the sophistication conundrum.
During our analysis, we paid particular attention to what we deemed to be sophisticated technical capabilities such as the ability to handle context switching, dealing with multiple intents, and the use of avatars. First, we found that most of the technology providers are not providing these advanced capabilities or do so in only a limited fashion.
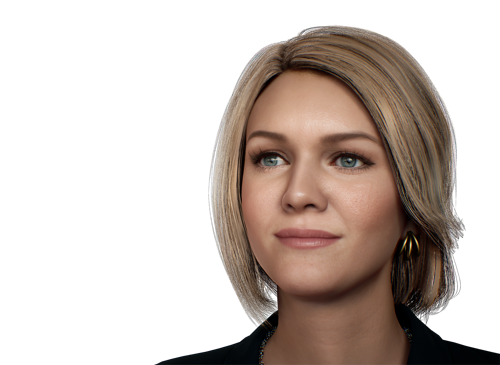
Amelia.ai's avatar. Source: Amelia.ai
What was most surprising, however, was that many providers stated that they were not supporting these more sophisticated capabilities at this point not because they lacked the technical ability to do so, but rather because of a lack of customer demand. (Note: we could not verify these undemonstrated claims and our analysis indicates that these sophisticated capabilities are not necessarily easy to deliver.)
Current customers of conversational AI systems, it would appear, are implementing them to solve relatively simple use cases such as advanced knowledgebase queries, product search, customer support, and employee assistance of routine tasks. Even among those enterprise who have purchased a solution that offered these sophisticated capabilities, use appears to be limited.
We suspect that we can attribute much of the situation here to a relative lack of maturity when it comes to customer usage of these conversational AI systems: we are all too accustomed to using ‘dumb bots’ to even attempt to leverage more sophisticated capabilities even if they exist. We further believe that this situation will change and will likely do so rapidly once customers become conditioned to more sophisticated use cases presented by consumer-oriented intelligent assistants.
Therefore, enterprise leaders should evaluate the more sophisticated capabilities with two eyes: one looking at current use case needs, and one toward future expectations. In doing so, we believe that investing in platforms that provide a pathway to more sophisticated use cases will likely be beneficial over time.
Evaluating & Selecting Your Conversational AI Partner
Given the diverse landscape of the market and the various use cases in which you can apply the technology, evaluating and selecting a conversational AI partner can be challenging. As you do so, it will be helpful to establish an evaluation framework.
While you should develop such an evaluation framework based on your own specific use case and needs, you should ensure that you are evaluating it in at least four dimensions:
Technical
Quality
Experiential
Ethical
Within each dimension, you should look at specific characteristics that will best help you evaluate it. Using this characteristic matrix will help you to evaluate appropriate partners for your situation.
We identified the following characteristics that we believe help in this process:
Note: Click each characteristic category to toggle it open.
Conversational AI Providers to Consider
While we do not compare or rate technology providers, we do evaluate them as to their fit as part of the ExTech Market. In so doing, we attempt to answer a single question: can this technology provider help enterprises create, deliver, and manage a differentiated digital experience?
This evaluation demands that they possess adequate levels of each of the characteristics presented above, and that they are sufficiently aligned in both their product strategy and go-to-market positioning with the core principles represented within the Digital Experience Value Engine.
As part of this research, we reached out to and briefed with numerous conversational AI providers. Those listed below met our criteria and we believe are viable options for any enterprise leader building a value engine and looking to leverage conversational AI as part of it, depending on their needs. If a technology provider is not listed, it means that either it did not meet our criteria or that it did not brief us.
Each technology provider is presented using the following notations:
Proprietary or Orchestration to denote whether or not it is exclusively or primarily leveraging proprietary technology or orchestrating available technologies.
Voice, Text or Dual Deployment Channel to denote which deployment channels it primarily supports.
Use Case:General or as specified to denote its target use case with ‘general’ meaning that the technology can be applied to all or most use cases.
Advanced, Intermediate or Standard indicates the degree to which it supports the most sophisticated use cases and features, however, all levels are sufficient to meet most current enterprise use cases.
Conversational AI Providers, listed alphabetically:
Technology:
Proprietary
Deployment:
Text
Use Case:
Customer Experience
Sophistication:
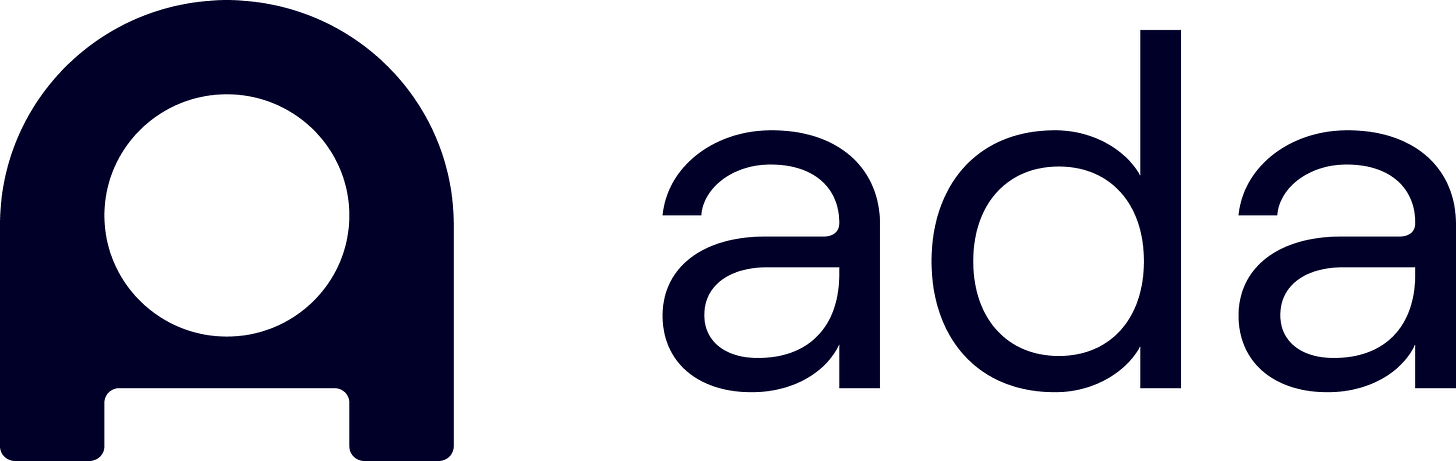
Make Everyone Feel Like a VIP. Ada is the automation layer that powers interactions between you and your customers, enabling your brand to be continuously available, completely consistent, and always helpful.
Technology:
Proprietary
Deployment:
Dual
Use Case:
General
Sophistication:
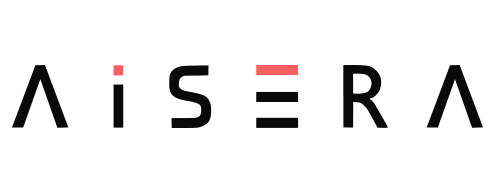
AI Service Experience. End-to-End Enterprise Service Automation. Aisera empowers organizations with business uptime, improved productivity, cost reduction, and a consumer-like self-service experience for both employees and customers.
Technology:
Proprietary
Deployment:
Dual
Use Case:
General
Sophistication:
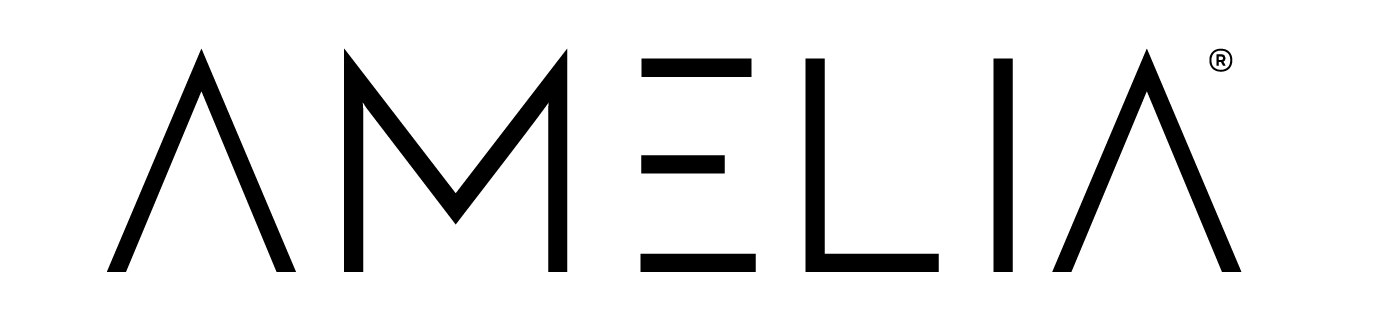
The Most Human AI. Purpose-built to help companies transform into friction-less digital enterprises utilizing Amelia’s Conversational AI and AIOps, enabled by foundational Orchestration Services. Customers leverage the Amelia competencies best suited for their specific use cases.
Technology:
Proprietary
Deployment:
Dual
Use Case:
Banking Customer Experience
Sophistication:
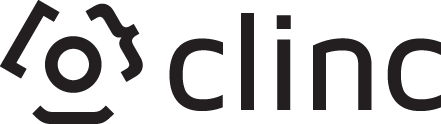
We hear you. Clinc’s revolutionary conversational AI has been proven successful at some of the largest banks worldwide. The best-in-class technology utilizes natural language processing that understands how people really talk, powering exceptional customer experiences.
Technology:
Proprietary
Deployment:
Text
Use Case:
General
Sophistication:
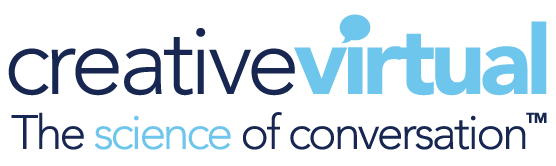
Bringing Together Humans and Artificial Intelligence. Deliver seamless, personalized digital support for your customers, employees, and contact center agents with our powerful conversational AI platform.
Technology:
Orchestrating
Deployment:
Text
Use Case:
IT Support
Sophistication:
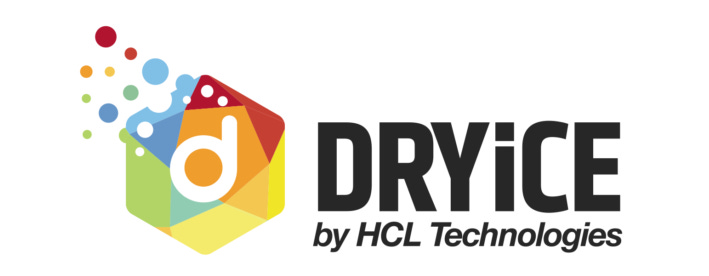
AI-enabled cognitive virtual assistant. In DRYiCE Lucy, you have a conversational artificial intelligence (AI) platform and a digital colleague that makes multi-turn conversations and offers superlative user experience.
Technology:
Proprietary
Deployment:
Dual
Use Case:
Employee Experience
Sophistication:
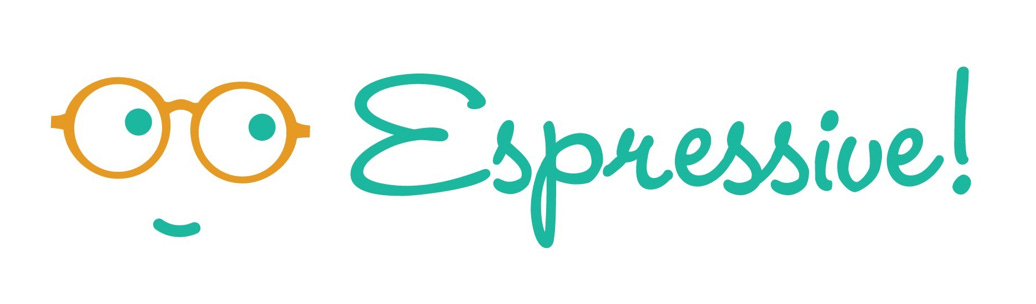
Frictionless Self-Help In a Work From Anywhere World. Espressive Barista, our AI-based virtual support agent (VSA), incorporates advanced NLP and conversational AI to automate resolution of employee questions, issues, and requests with personalized responses that result in elimination of 70% of help tickets, enabling you to recoup 1.2 weeks of productivity per employee per year.
Technology:
Orchestration
Deployment:
Dual
Use Case:
Customer Experience
Sophistication:
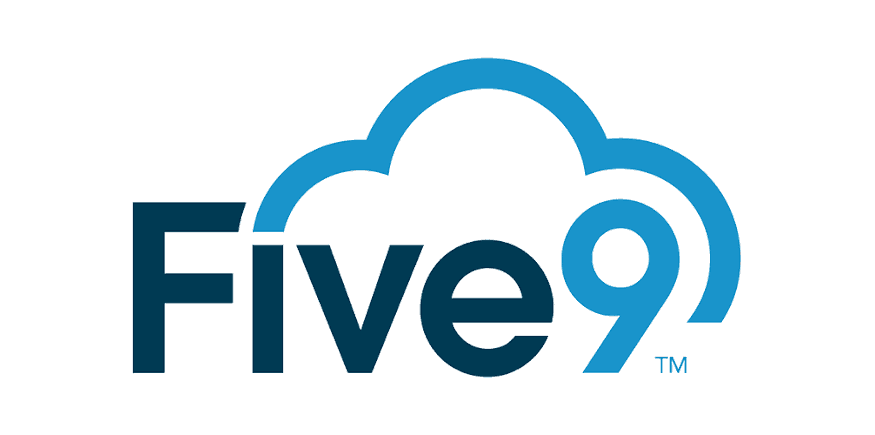
Virtual Agents Deliver the Experience Customers Want. Automate routine and repetitive tasks with Five Intelligent Virtual Agent so your live agents can focus on higher value work. Five9 IVA uses practical AI technology to understand what customers want and provide it quickly—without the use of a human agent.
Technology:
Proprietary
Deployment:
Dual
Use Case:
Customer Experience
Sophistication:
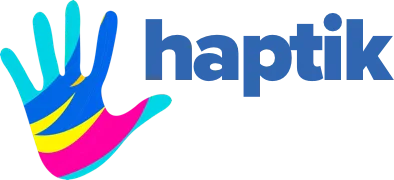
Driving the World’s Transition to AI-Powered Conversations. Build powerful Intelligent Virtual Assistants with Haptik’s Enterprise CX platform. Amplify engagement, increase conversions, and deliver delightful support to your customers.
Technology:
Proprietary
Deployment:
Text
Use Case:
Customer Experience
Sophistication:
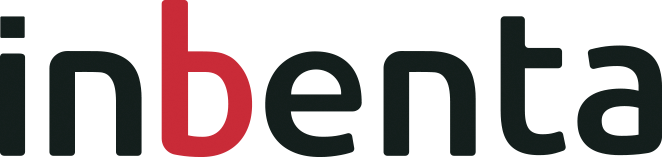
An easy-to-use AI platform to build seamless customer interactions. Reduce handling costs & boost digital self-service. Increase conversion and engagement rates across all channels.
Technology:
Proprietary
Deployment:
Dual
Use Case:
General
Sophistication:
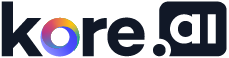
Engage your customers and employees in meaningful conversations with Kore.ai’s industry-leading, AI-first conversational interface. Accelerate your development with purpose-built, domain-trained, highly customizable assistants for voice and digital channels.
Technology:
Orchestration
Deployment:
Dual
Use Case:
Customer Experience
Sophistication:
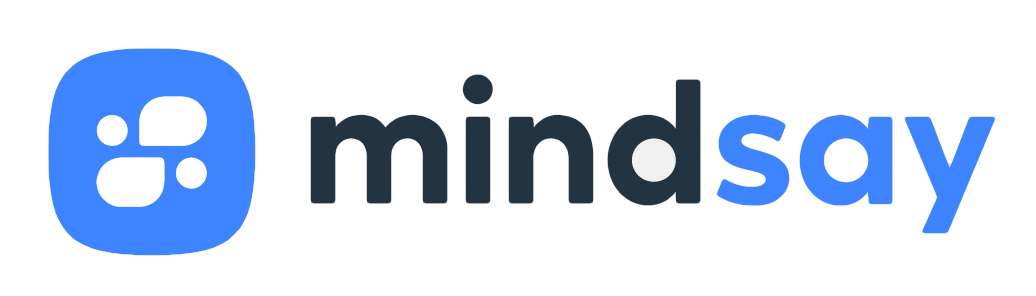
Simple and efficient customer interactions. Deliver exceptional customer self-service with Mindsay's no-code conversational AI platform.
Interested in licensing this report? Contact us at info@digitalexperiencereport.com.
Sources
Capgemini (October 26, 2018). Artificial intelligence and conversational user interfaces powering customer experience. https://www.capgemini.com/2018/10/artificial-intelligence-and-conversational-user-interfaces-powering-customer-experience/. Accessed October 12, 2020
Deloitte (2020). Conversational AI - The next wave of customer and employee experiences Available: https://www2.deloitte.com/content/dam/Deloitte/au/Documents/strategy/au-deloitte-conversational-ai.pdf. Accessed: September 28, 2020.
Digital Reality (April 9, 2020). Customer Experience Relies on IT Infrastructure. https://www.digitalrealty.com/blog/customer-experience-relies-on-it-infrastructure.
Ezekiel, K., Vassilev, V., Ouazzane, K., & Patel, Y. (2019). Adaptive business rules framework for workflow management. Business Process Management Journal.
Forbes (Aug 17, 2017). The Evolution and Future of AI in Conversational Commerce and Customer Experience.https://www.forbes.com/sites/briansolis/2017/08/17/the-evolution-and-future-of-ai-in-conversational-commerce-and-customer-experience/#1856bfc24c90. Accessed October 12, 2020.
Forbes (Oct 5, 2020). What Is GPT-3 And Why Is It Revolutionizing Artificial Intelligence? https://www.forbes.com/sites/bernardmarr/2020/10/05/what-is-gpt-3-and-why-is-it-revolutionizing-artificial-intelligence/#68b92ea0481a. Accessed October 12, 2020.
Gnewuch, U., Morana, S., & Maedche, A. (2017, December). Towards Designing Cooperative and Social Conversational Agents for Customer Service. In ICIS.
Graham, D., & Bachmann, T. T. (2004). Ideation: The birth and death of ideas. John Wiley & Sons.
Granito, F.A., (2020). Are you ready for transformation? The Institute for Digital Transformation.
Grewal, D., Levy, M., & Kumar, V. (2009). Customer experience management in retailing: An organizing framework. Journal of retailing, 85(1), 1-14.
Harvard Business Review (September 23, 2020). The Next Big Breakthrough in AI Will Be Around Language. https://hbr.org/2020/09/the-next-big-breakthrough-in-ai-will-be-around-language?utm_medium=email&utm_source=newsletter_weekly&utm_campaign=weeklyhotlist_not_activesubs&deliveryName=DM98953. Accessed October 12, 2020.
Hessey, S., & Venters, W. (2016, July). Sensing distress–towards a blended method for detecting and responding to problematic customer experience events. In International Conference on HCI in Business, Government, and Organizations (pp. 395-405). Springer, Cham.
Jacobs, Randy, Evaluating Satisfaction with Media Products and Services: An Attribute Based Approach, European Media Management Review, Winter 1999. http://www.tukkk.fi/mediagroup/emmr/Previous%20Issues/Satisfaction.htm
Jadeja, M., & Varia, N. (2017). Perspectives for evaluating conversational AI. arXiv preprint arXiv:1709.04734.
Johansson, T., & Kidron, T. (2017). Improving Customer Experience in Telecommunications Company.
Kauko, J. (2018). Improving Operations towards ISO/IEC 20000 Certification for A Cloud SaaS Provider: On the example of Capacity Management process and Process manager roles.
Khwaja, A., & Yang, N. (2018). Quantifying the Link Between Employee Engagement and Customer Satisfaction in the Car Rental Industry. Available at SSRN 3213659.
Liedtka, J. (2014). Innovative ways companies are using design thinking. Strategy and Leadership, 42(2), 40-45.
Lilja, A., & Kihlborg, M. (2020). Important criteria when choosing a conversational AI platform for enterprises.
Mankins, M. (2014). This weekly meeting took up 300,000 hours a year. Harvard Business Review, 29.
Martin, R. L. (2017). Strategic choices need to be made simultaneously, not sequentially. Harvard Business Review.
McKinsey (June 22, 2020). Technology and innovation: Building the superhuman agent. Need login: https://www.mckinsey.com/business-functions/operations/our-insights/technology-and-innovation-building-the-superhuman-agent. Accessed October 12, 2020.
Meyer, C., & Schwager, A. (2007). Understanding customer experience. Harvard business review, 85(2), 116.
Mosweu, O. E. (2017). An assessment of the capacity management process of the information technology infrastructure library (ITIL) framework in delivering value in public sector (Doctoral dissertation, Cape Peninsula University of Technology).
Parise, S., Guinan, P. J., & Kafka, R. (2016). Solving the crisis of immediacy: How digital technology can transform the customer experience. Business Horizons, 59(4), 411-420.
Park, S., Li, H., Patel, A., Mudgal, S., Lee, S., Kim, Y. B., ... & Sarikaya, R. (2020). A Scalable Framework for Learning From Implicit User Feedback to Improve Natural Language Understanding in Large-Scale Conversational AI Systems. arXiv preprint arXiv:2010.12251.
Porter, M.E. (2005). What is Strategy. Harvard business review, 2607.
Pirovano, A. (2018). State of art and best practices in generative conversational AI.
Rajkumar, S. (2010). Art of communication in project management. Paper presented at PMI® Research Conference: Defining the Future of Project Management, Washington, DC. Newtown Square, PA: Project Management Institute.
Ruane, Elayne & Birhane, Abeba & Ventresque, Anthony. (2019). Conversational AI: Social and Ethical Considerations.
Safin, S., Dorta, T., Pierini, D., Kinayoglu, G., & Lesage, A. (2016). Design Flow 2.0, assessing experience during ideation with increased granularity: A proposed method. Design Studies, 47, 23-46.
Spiess, J., T'Joens, Y., Dragnea, R., Spencer, P., & Philippart, L. (2014). Using big data to improve customer experience and business performance. Bell labs technical journal, 18(4), 3-17.
Sull, D., Turconi, S., Sull, C., & Yoder, J. (2018). Turning strategy into results. MIT Sloan Management Review.
Ullman, David G., The Mechanical Design Process, McGraw-Hill, Inc., U.S.A., 1997 pp. 105-108 ISBN 0-07-065756-4
Venkatesan, R. (2017). Executing on a customer engagement strategy.
Vivek, S. D., Beatty, S. E., & Morgan, R. M. (2012). Customer engagement: Exploring customer relationships beyond purchase. Journal of marketing theory and practice, 20(2), 122-146.
Vivek, S. D., Beatty, S. E., Dalela, V., & Morgan, R. M. (2014). A generalized multidimensional scale for measuring customer engagement. Journal of Marketing Theory and Practice, 22(4), 401-420.